Can Emotional AI Truly Understand Human Emotions? A Deep Dive into Sentiment Analysis
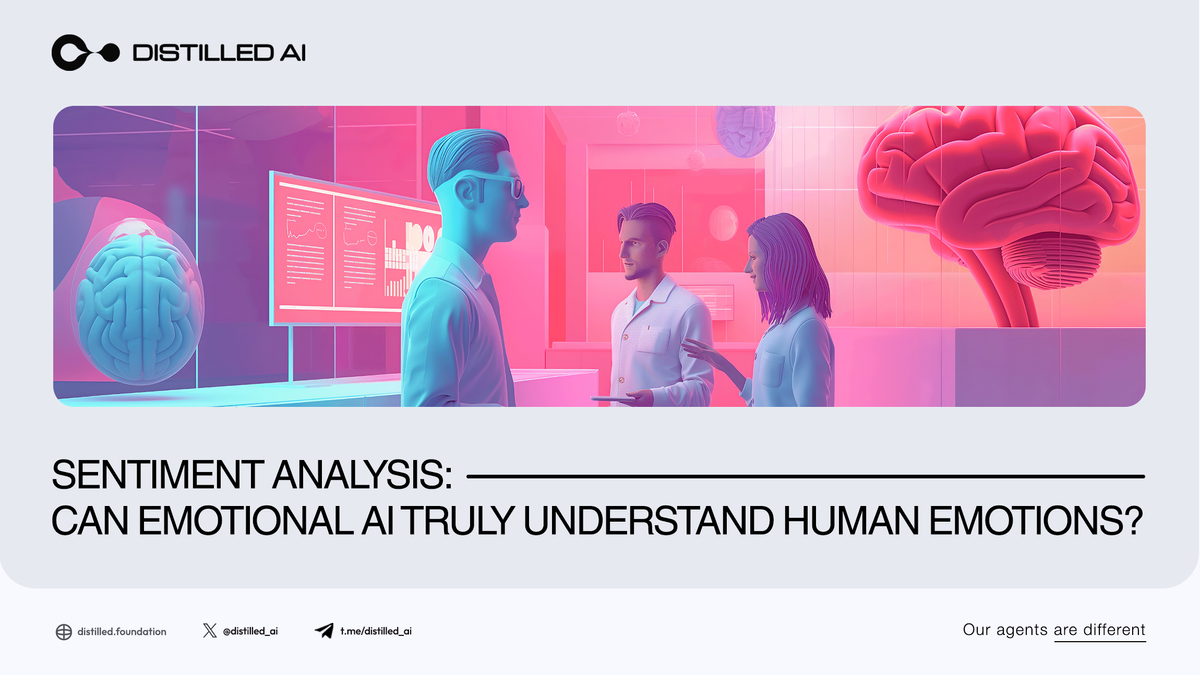
Introduction
Emotional AI—often dubbed affective computing—promises machines that can detect, interpret, and even respond to human emotions. But can these AI systems really grasp the full nuance of human sentiment? The linchpin of emotional AI is sentiment analysis, an area where machine learning and natural language processing meet. Here, we take a deep dive into how sentiment analysis works, its accuracy, and what experts say about AI’s capability to truly understand human emotions.
1. How Sentiment Analysis Works
Core Techniques
• Lexicon-Based Methods: Use predefined lists of positive, negative, or neutral words to gauge the sentiment of text.
• Machine Learning: Trains algorithms on vast labeled datasets to identify sentiment patterns. Neural networks or transformer models (e.g., BERT, GPT) have boosted performance.
• Contextual Parsing: Modern systems also factor in emoji, punctuation, and cultural references to interpret sentiment more precisely.
Data Sources
Social media posts, online reviews, and customer support transcripts feed these models, helping them recognize emotion in diverse contexts.
Why It Matters: Effective sentiment analysis enables AI to offer empathetic responses—crucial for mental health chatbots, customer service interactions, and social media monitoring.
2. Accuracy and Limitations
Accuracy Benchmarks
Recent research shows state-of-the-art models can surpass 90% accuracy on benchmark datasets—impressive, yet not foolproof. Text complexities (sarcasm, irony, slang) pose ongoing challenges.
Contextual Gaps
Users’ cultural norms and personal expression styles can significantly affect sentiment interpretation. Without broader context, AI might misread emotional cues, leading to false positives or negatives.
Ambiguity and Nuance
Human language brims with subtleties (e.g., rhetorical questions, double meanings). Even advanced AI can misinterpret a user’s tone if the text is laden with sarcasm or self-deprecating humor.
Takeaway: While sentiment analysis has made remarkable strides, AI’s ability to truly “feel” or comprehend deep emotional subtext remains an evolving frontier.
3. Research Findings and Expert Insights
Peer-Reviewed Studies
• A study in Computational Linguistics found BERT-based models excel in “straightforward” sentiments but lag on ambivalent or mixed tones.
• Research at MIT indicates adding multi-modal data (facial expressions, vocal cues) can increase accuracy by up to 15% over text-only methods.
Expert Opinions
• Dr. Alisha Grant (NLP researcher): “AI can approximate emotional understanding through patterns, but genuine empathy remains a purely human domain—for now.”
• Paul Stevens (AI ethicist): “Context is king. Emotion is not just in the text, but in human relationships and cultural undercurrents.”
Result: These perspectives highlight the potential and the caveats of relying heavily on sentiment analysis for true emotional comprehension.
4. Balancing Expectations and Reality
Key Use Cases
• Customer Support: AI chatbots quickly detect negative sentiment and escalate conversations to human agents.
• Brand Reputation Management: Monitoring large-scale social media sentiment offers real-time feedback loops.
• Mental Health Applications: Early-warning detection of depression or anxiety through textual clues in self-expressions.
Practical Limitations
Despite robust analytics, these applications must incorporate human oversight—particularly in sensitive arenas (e.g., therapy, crisis hotlines). AI’s inability to interpret full emotional context calls for a hybrid approach where machine efficiency supports, rather than replaces, human empathy.
Bottom Line: Current sentiment analysis tools can offer tremendous value, but relying on them as definitive emotional interpreters oversimplify human emotional complexity.
5. The Future of Emotional AI
Multimodal Synthesis
Next-gen emotional AI aims to combine voice analysis, facial recognition, and physiological data with textual sentiment for a more holistic emotional profile.
Personalization
Systems may soon adapt to individual emotional expression styles—improving accuracy over time. AI agents might learn that one user’s sarcastic remarks are actually affectionate banter, adjusting sentiment evaluations accordingly.
Ethical Focus
As emotional AI becomes more accurate, ethics and privacy debates intensify. Ensuring user consent, data protection, and transparent AI decision-making will remain central to responsible deployment.
Conclusion
So, can emotional AI truly understand human emotions? Sentiment analysis—the bedrock of emotional AI—continues to improve, providing valuable insights into human expressions. Yet even advanced systems can stumble on cultural nuances, irony, and deep emotional complexity. Experts agree we’re far from achieving machine-based empathy that equals human-level understanding. In practice, balancing technological capabilities with human oversight remains paramount to avoid misinterpretations—and to harness AI’s potential for good in diverse fields, from customer service to mental health.
Key Takeaways
1. Evolving Accuracy: AI models can surpass 90% on straightforward sentiment tasks but struggle with nuanced emotion.
2. Context is Critical: Sarcasm, cultural references, and personal expression styles can confuse even advanced NLP systems.
3. Expert Consensus: While AI is adept at pattern recognition, true emotional empathy remains a human domain.
4. Hybrid Future: Combining AI’s efficiency with human judgment can yield safer, more empathetic applications.
By maintaining realistic expectations and robust ethical frameworks, emotional AI can continue driving innovation—while acknowledging human emotions aren’t fully captured in mere data points.