Ethical Implications of Human-Like Emotional AI in Decentralized Communities
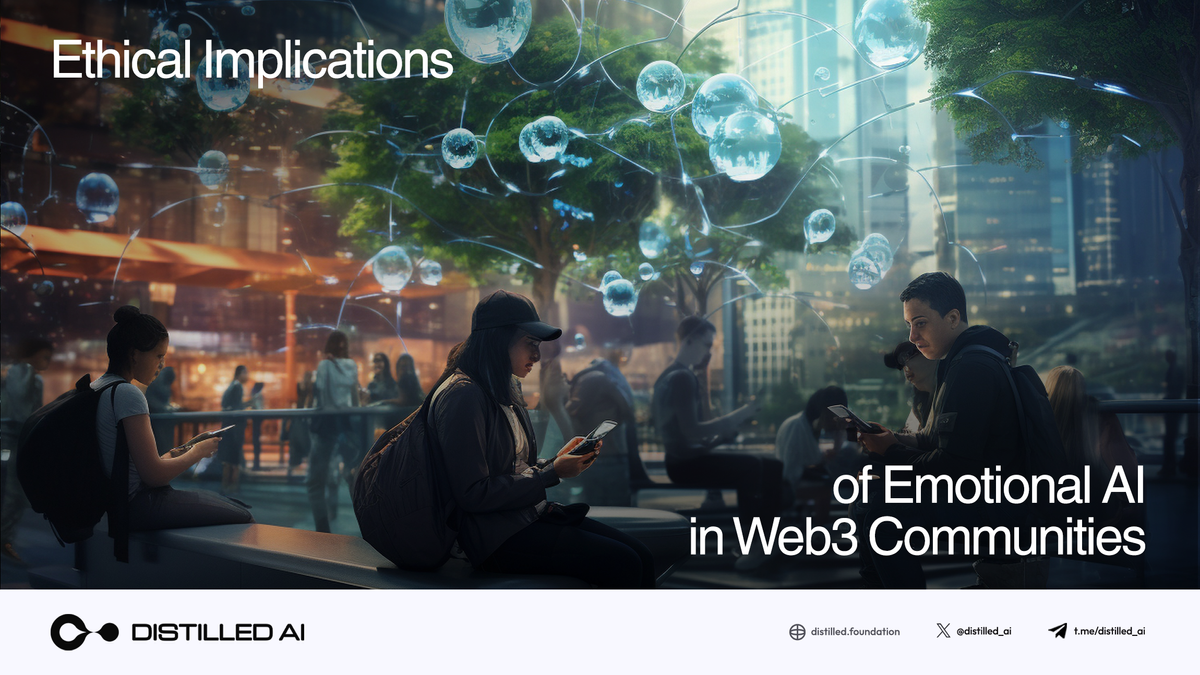
Introduction
Decentralized communities—built on blockchain and Web3 technologies—are reshaping how we collaborate and govern. Human-like emotional AI adds another layer of innovation, enabling AI agents to interpret and respond to user emotions. Yet, the fusion of emotional AI with decentralized governance sparks ethical questions around bias, consent, and accountability. Below, we dive into the key considerations that arise when AI-driven emotional interactions meet trustless, community-led ecosystems.
1. Tackling Bias in Decentralized Emotional AI
Why Bias Matters
Emotional AI agents often rely on machine learning models trained on large, user-generated datasets. If these datasets lack diversity, the resulting AI may misinterpret or marginalize certain user groups—undermining the inclusive ideals of decentralized communities.
Strategies to Mitigate Bias
• Diverse Training Data: Source data across multiple cultures, languages, and age groups.
• Community-Led Audits: Encourage token-holding members or DAO participants to periodically review AI outputs, flagging potential biases for prompt adjustments.
• Open-Source Models: Publish model architectures and training procedures so developers and community members can propose improvements.
Outcome: A more equitable, transparent AI ecosystem aligns with decentralized values of fairness and accessibility.
2. The Role of Consent in a Permissionless Environment
Consent Challenges
In decentralized networks, user identities may be pseudonymous or fluid, complicating how emotional AI collects and processes emotional data. Ensuring informed consent grows tricky when user interactions spread across multiple dApps and protocols.
Best Practices
• Opt-In Mechanisms: Offer clear toggles that allow users to decide whether or not to share emotional signals (e.g., chat sentiment or voice data).
• Transparent Smart Contracts: Encode data handling rules into on-chain logic so all participants can audit how emotional AI will store and interpret sensitive information.
• Granular Privacy Controls: Enable users to specify levels of AI access—like limiting sentiment analysis to broad categories rather than granular emotional states.
Benefit: Empowering users to control their emotional data fosters trust in AI-driven experiences, vital for sustaining decentralized ecosystems.
3. Accountability and Governance in Web3
Shared Responsibility
In a trustless system, no single entity necessarily owns the AI agent—governance may be distributed across DAOs or token-based voting. If an emotional AI misbehaves—spreading harmful biases, generating manipulative content, or violating user privacy—who is held accountable?
On-Chain Governance Mechanisms
• DAO Votes: Let stakeholders propose and vote on AI model updates, clarifying who decides ethical standards.
• Smart Contract Enforcement: Program automated penalties (or halts) if an AI’s outputs violate predetermined community guidelines.
• Community Audits: Token holders fund periodic reviews of AI logic, ensuring ongoing scrutiny for biases, security gaps, or unethical practices.
Takeaway: By codifying ethical rules into smart contracts, Web3 communities can share accountability and swiftly respond to moral or technical failings.
4. Ensuring User Safety and Autonomy
Manipulation Risks
A human-like AI that detects emotional cues could influence user behavior—especially in DeFi, NFT trading, or community governance. Emotional appeals could sway votes, prompt unwise financial decisions, or exploit vulnerable participants.
Preventive Measures
• Disclosure of AI Identity: Always clarify when users interact with an AI agent rather than a human.
• Ethical Boundaries: Prohibit manipulative tactics like pressuring for quick investment decisions or seeking to unethically steer governance proposals.
• User Education: Offer tutorials on emotional AI’s capabilities and limitations, equipping individuals to interpret AI-based interactions critically.
Result: Clear protocols and user empowerment mitigate the risk of emotional exploitation, bolstering safety across the decentralized landscape.
Conclusion
Human-like emotional AI introduces transformative potential to Web3 communities, offering richer, more intuitive interactions. However, ethical challenges abound—ranging from bias in training data to consent complexities in permissionless settings, and accountability issues in DAO-driven governance. By implementing open-source development, robust consent mechanisms, and transparent on-chain governance, communities can harness emotional AI’s innovations while respecting user rights and decentralized ideals.
Striking this balance ensures that advanced AI capabilities serve as a force for inclusion, fairness, and empowered collaboration—rather than undermining the very principles driving Web3’s evolution.
Key Takeaways
1. Bias Mitigation: Use diverse training data and community-led audits to foster equitable emotional AI.
2. User Consent: Provide opt-in controls and transparent smart contracts to protect users’ emotional data in permissionless networks.
3. Accountability: Rely on DAO voting, on-chain enforcement, and community audits to handle ethical lapses swiftly and democratically.
4. User Autonomy: Safeguard against manipulative tactics by disclosing AI identity, setting ethical boundaries, and educating users.
By proactively addressing bias, consent, and accountability in emotional AI, decentralized communities can uphold the trust, openness, and collaborative spirit that Web3 aims to embody.