LLMs and DeFi: Automating Financial Predictions in Decentralized Markets
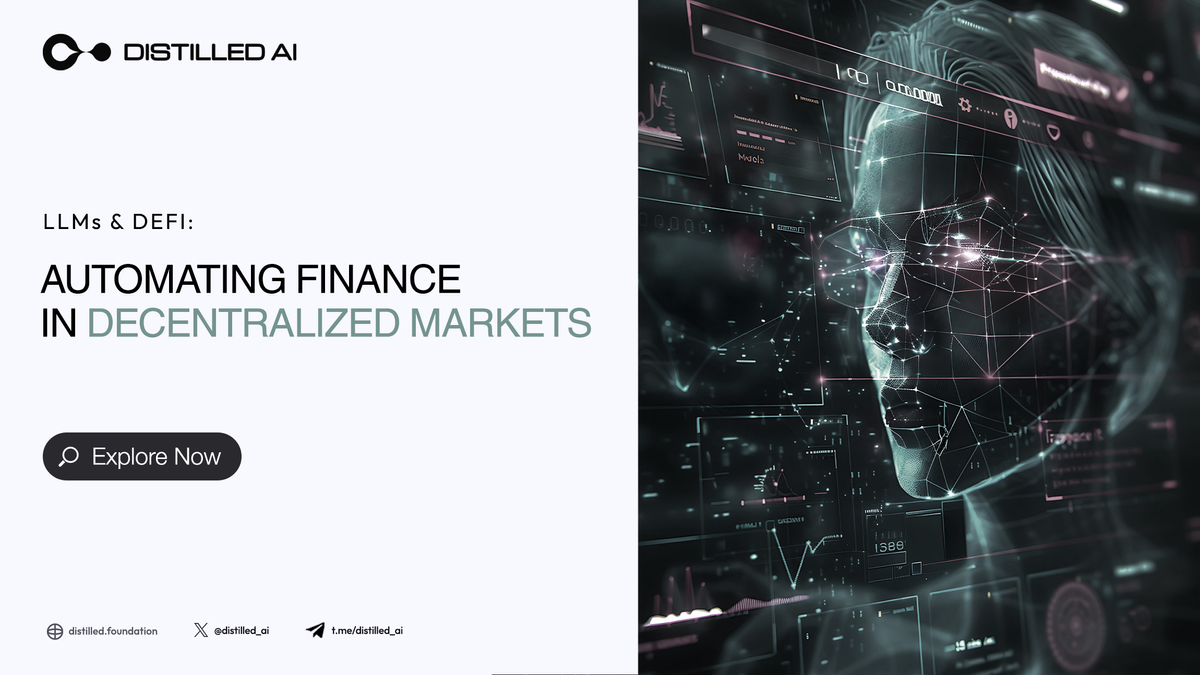
Decentralized Finance (DeFi) is reshaping the financial sector by empowering users to transact, invest, and manage assets on trustless, blockchain-driven platforms. At the same time, Large Language Models (LLMs) have emerged as powerful AI tools for data analysis and automation. When applied to DeFi, LLMs open up new possibilities for predictive analytics, risk assessment, and algorithmic trading strategies. Below, we delve into how LLMs can optimize financial predictions and drive innovation in Decentralized Markets.
1. Data-Driven Predictive Analytics
Why LLMs?
LLMs can process massive volumes of unstructured data—think social media sentiment, news articles, and community chats—to extract market signals. This contextual understanding helps DeFi participants anticipate price movements, token demand, or liquidity shifts.
Market-Wide Surveillance
Unlike traditional algorithms limited by static data feeds, LLMs interpret real-time textual information for holistic market insights. This capability is especially useful in volatile DeFi markets, where an influencer’s tweet or governance proposal can rapidly move asset prices.
Takeaway: By scanning and synthesizing decentralized ecosystems, LLMs generate actionable insights for more informed investment decisions.
2. Enhancing Risk Assessment
Identifying Protocol Vulnerabilities
LLMs can assist in auditing DeFi smart contracts by spotting common coding pitfalls or potential exploit patterns. They also help interpret on-chain data—like token flows or user interactions—to detect abnormal behavior that might signal a hack or market manipulation attempt.
Automated Credit Scoring
DeFi lending protocols often rely on collateral rather than creditworthiness. However, advanced systems can integrate LLM-powered sentiment or historical transaction analysis to gauge borrower reliability. This approach refines existing collateral requirements, enabling more flexible lending terms and lower liquidation risks.
Result: AI-driven risk assessments enhance transparency and confidence, fueling mainstream DeFi adoption with safer protocols and loans.
3. Algorithmic Trading and Strategy Automation
Sentiment-Aware Bots
AI trading bots use LLMs to track crypto news, forum discussions, or DAO proposals—reacting instantly to market sentiment. By factoring in user emotions or trending topics, these strategies potentially outperform purely technical analyses.
Smart Yield Optimization
DeFi offers yield farming options, where users stake tokens across pools or vaults for returns. LLMs can continually evaluate variables—like APY shifts, smart contract changes, and asset correlations—to optimize deposit allocations on the fly, minimizing human oversight.
Outcome: Automated strategies powered by real-time textual intelligence allow traders and liquidity providers to capitalize on rapid DeFi market fluctuations.
4. Balancing Trustlessness with AI Insights
Decentralized Oracles
To maintain a trustless environment, DeFi applications rely on oracles for off-chain data. Integrating LLM outputs into these oracles can raise concerns about authenticity and potential manipulation. Transparent governance frameworks and cryptographic verification ensure the LLM’s predictions do not introduce single points of failure.
DAO Governance
LLMs can parse complex proposals and explain potential impacts to token holders in user-friendly summaries. This synergy enables informed community decisions—like updating collateral ratios or adding new token pairs—without undermining the decentralized ethos.
Key Insight: Aligning LLM-driven intelligence with DeFi’s trustless architecture requires robust on-chain verification and community oversight.
5. Challenges and Future Outlook
Model Accuracy & Overfitting
LLMs risk producing false positives if they overfit to niche data patterns or lack updated training sets. Constant model validation and periodic fine-tuning are crucial for sustained performance in fast-evolving DeFi markets.
Ethical and Regulatory Concerns
Automated strategies can influence token prices or create flash liquidation events, raising issues around market manipulation or unfair advantage. Proactive compliance with emerging DeFi regulations and transparent AI practices will be pivotal.
Emerging Trends
As DeFi expands to cross-chain ecosystems, LLMs will need to parse multi-protocol data—from Ethereum to Cosmos—to produce unified analytics. Multi-modal integration (e.g., analyzing text, on-chain logs, and transaction graphs together) hints at the next frontier for AI-augmented DeFi solutions.
The convergence of Large Language Models and Decentralized Finance elevates how market predictions, risk management, and automated strategies are developed. From sentiment analysis to advanced smart contract audits, LLMs empower DeFi participants to navigate volatile crypto markets with agility and confidence. However, the success of these integrations hinges on addressing trust and transparency within Web3’s trustless frameworks. With ongoing technological refinement and community-driven governance, AI-infused DeFi stands to redefine what’s possible in the realms of finance, innovation, and digital autonomy.
Key Takeaways
1. Data-Driven Predictions: LLMs interpret large text-based datasets—social media, forums—to anticipate DeFi trends.
2. Risk Mitigation: Advanced AI audits and sentiment analyses help identify protocol vulnerabilities, shaping safer lending options.
3. Automated Strategies: Bot-driven trades and yield optimizations capitalize on real-time market insights, reducing human intervention.
4. Ethical & Regulatory Balance: Avoiding single points of failure and over-reliance on AI predictions is critical for trustless DeFi.
5. Future Potential: Cross-chain analysis and multi-modal AI present new ways for DeFi projects to evolve in an expanding Web3 landscape.
By incorporating LLMs into DeFi workflows, the crypto community can forge intelligent and secure markets—merging AI’s computational prowess with the open, decentralized spirit of Web3.