Multi-Agent Collaboration in Team Communication: How AI Agents Enhance Messaging Platforms
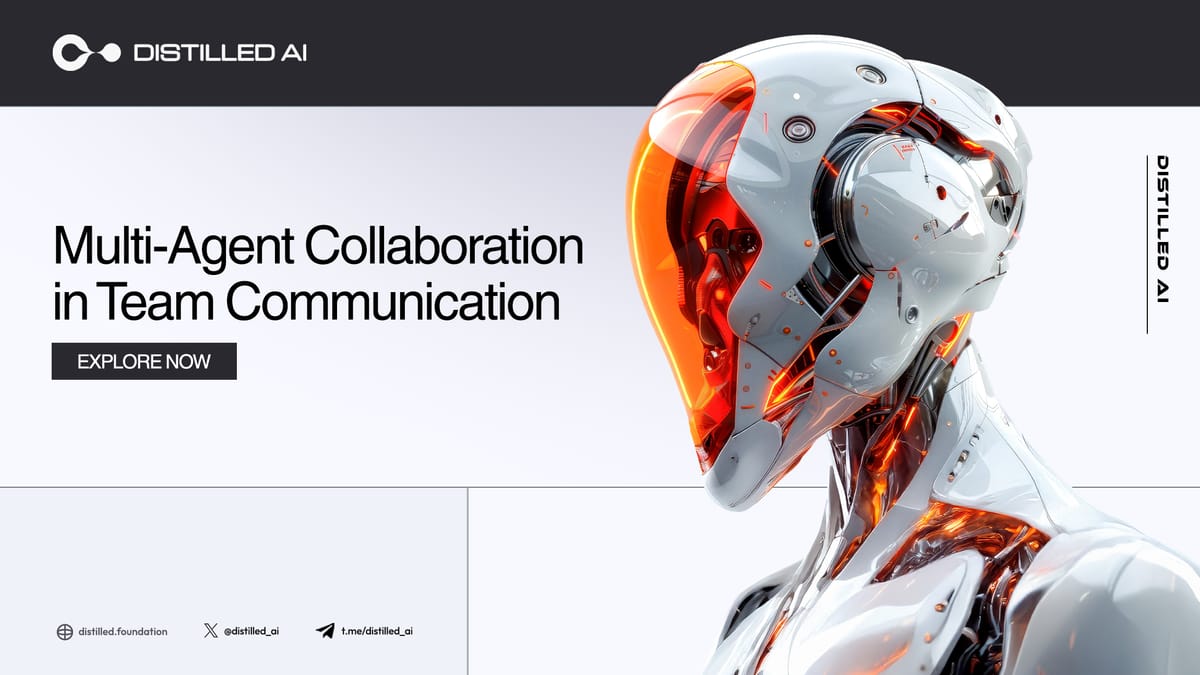
In today’s fast‐paced business landscape, seamless communication and collaboration are critical for success. With teams often distributed across various locations and time zones, organizations are increasingly turning to artificial intelligence (AI) to optimize internal communication. Multi-agent collaboration—where multiple AI agents work together—has emerged as a transformative solution. This article explores how AI agents enhance team communication within messaging platforms, driving efficiency, innovation, and better decision-making.
Understanding Multi-Agent Collaboration
Multi-agent collaboration refers to the use of several AI-powered agents that interact, coordinate, and share data to achieve common communication and operational goals. Unlike traditional single-agent systems, multi-agent frameworks mimic real-world teamwork by distributing tasks across specialized agents. Each agent can process distinct types of information, analyze trends, or monitor conversations in real time.
Key Features:
- Specialized Capabilities: Different agents can focus on distinct aspects of communication—from language translation and sentiment analysis to task management.
- Real-Time Data Processing: AI agents continuously monitor messaging platforms to extract insights, ensuring that communication remains dynamic and responsive.
- Interoperability: Agents can share information seamlessly, ensuring that data collected in one part of the system informs decisions in another.
By distributing responsibilities, multi-agent systems reduce bottlenecks and foster a more resilient communication ecosystem.
How AI Agents Work Together in Messaging Platforms
The Architecture Behind Multi-Agent Systems
At the heart of these systems lies a robust architecture based on Large Language Models (LLMs) and modular design principles. The architecture can be broken down into several core components:
- Core/Brain: The “brain” of each AI agent is powered by advanced LLMs—such as GPT-4—that generate human-like text and understand context. This core adapts its language style and tone to match organizational requirements.
- Planning Mechanisms: AI agents employ both feedback-independent and feedback-based planning. They generate initial content drafts or action plans, then refine these outputs using real-time analytics and user feedback.
- Memory Systems:
- Short-Term Memory (STM): Allows agents to retain context over a conversation or a task session.
- Long-Term Memory (LTM): Stores historical data and interactions, ensuring continuity and enabling personalization.
- Tools and Integrations: AI agents leverage external APIs, retrieval-augmented generation pipelines, and integration with enterprise systems (like CRM and project management tools) to pull in data that enriches the conversation.
Seamless Interaction in Messaging Platforms
When integrated with messaging platforms (such as Slack, Microsoft Teams, or custom enterprise chat systems), AI agents can:
- Monitor Conversations: Automatically scan chat channels for keywords, detect sentiment, and flag urgent issues.
- Provide Real-Time Assistance: Offer instant suggestions, schedule meetings, and summarize long threads, reducing information overload.
- Automate Routine Tasks: Handle recurring requests (like setting reminders, sharing documents, or updating task lists) so team members can focus on strategic activities.
- Translate and Adapt Communication: Break down language barriers by providing real-time translations, ensuring global teams remain on the same page.
Enhancing Team Communication: Practical Benefits
Improved Efficiency and Productivity
Multi-agent collaboration streamlines workflow by automating repetitive tasks. For instance, an AI agent can draft meeting summaries while another integrates feedback from various departments—all within a single messaging thread. This automation significantly reduces manual work, speeds up communication, and ensures that key information isn’t lost in long conversations.
Data-Driven Insights
AI agents continuously analyze chat data to identify trends and bottlenecks. By leveraging real-time analytics, they can:
- Detect Communication Gaps: Identify topics that need further clarification or areas where team members repeatedly ask similar questions.
- Predict Project Risks: Highlight potential delays or misunderstandings early, allowing managers to intervene proactively.
- Enhance Decision-Making: Provide actionable insights based on historical data, ensuring that teams make informed decisions quickly.
Enhanced Collaboration and Cross-Functional Integration
By acting as digital intermediaries, AI agents bridge the gap between different departments. For example:
- Content and Technical Teams: While content teams focus on creative messaging, technical agents ensure that the content is optimized for internal knowledge bases and meets digital communication standards.
- Remote Teams: In distributed work environments, AI agents help maintain consistency and alignment by continuously updating shared dashboards and collaborative documents.
Implementation Strategies for Businesses
Implementing a multi-agent collaboration system involves both strategic planning and technical integration. Here are some best practices:
1. Define Clear Objectives
Before deployment, clearly outline what you expect to achieve:
- Enhance Efficiency: Set measurable goals such as reducing meeting times or improving response rates in internal communications.
- Improve Engagement: Focus on metrics like team satisfaction and the number of actionable insights generated by the AI.
2. Choose the Right Tools and Platforms
Select messaging platforms that support robust integrations with AI agents. Look for:
- API Accessibility: Platforms with open APIs make it easier to integrate custom AI solutions.
- Scalability: Ensure the platform can handle increased data flow and user interactions as your organization grows.
3. Foster a Collaborative Environment
Encourage team members to work alongside AI agents. This involves:
- Training Sessions: Educate teams on how to interact with AI tools effectively.
- Feedback Loops: Establish channels for employees to provide feedback on AI performance, allowing continuous improvement.
4. Monitor and Optimize
Regularly review system performance using analytics dashboards. Key performance indicators (KPIs) might include:
- Response Times: Measure how quickly AI agents address queries.
- User Engagement: Track the usage frequency and satisfaction levels.
- Operational Impact: Assess improvements in productivity and reductions in communication delays.
Future Trends in Multi-Agent Collaboration
As AI technology evolves, so too will the capabilities of multi-agent collaboration systems. Here are a few trends to watch:
Hyper-Personalization
Future AI agents will deliver even more personalized interactions by leveraging deeper insights into individual work habits and preferences. This will further tailor communications to each team member’s needs, enhancing productivity and satisfaction.
Real-Time Adaptability
Next-generation AI agents will be even more adaptive, capable of modifying their actions based on real-time changes in conversation dynamics and external data sources. This could lead to truly dynamic work environments where AI anticipates needs before they arise.
Increased Integration Across Tools
Expect tighter integrations between messaging platforms, project management tools, and enterprise resource planning systems. This unified approach will provide a holistic view of team operations, further enhancing collaboration and decision-making.
Ethical and Transparent AI
With increased reliance on AI in the workplace, there will be a growing emphasis on transparency and ethical guidelines. Future systems will clearly indicate AI-generated suggestions and ensure compliance with data privacy standards, building trust across the organization.
Challenges and Considerations
While the benefits of multi-agent collaboration are substantial, businesses must address potential challenges:
- Bias and Data Quality: AI agents rely on quality data. Ensure your training data is diverse and unbiased to prevent skewed outputs.
- Integration Complexity: Combining AI with existing communication tools can be technically challenging. Collaborate with IT experts and choose platforms with robust support.
- User Adoption: Resistance from team members can hinder success. Emphasize training and the tangible benefits of AI to foster a positive attitude towards these tools.
- Security Concerns: As AI agents handle sensitive internal communications, robust security protocols and compliance with data privacy regulations are essential.
Conclusion
Multi-agent collaboration in team communication represents a major step forward in leveraging AI to enhance internal workflows. By integrating specialized AI agents into messaging platforms, businesses can streamline operations, boost productivity, and foster a more engaged, data-driven culture. As organizations continue to navigate the digital transformation, those that adopt and refine multi-agent collaboration strategies will be better positioned to meet the challenges of tomorrow.
Implementing these technologies today not only improves communication efficiency but also sets the foundation for a more agile, innovative, and resilient organization. The future is collaborative—let AI agents be the catalyst that drives your team’s success.