Regulating Emotional AI in Web3: Balancing Innovation with User Safety and Trust
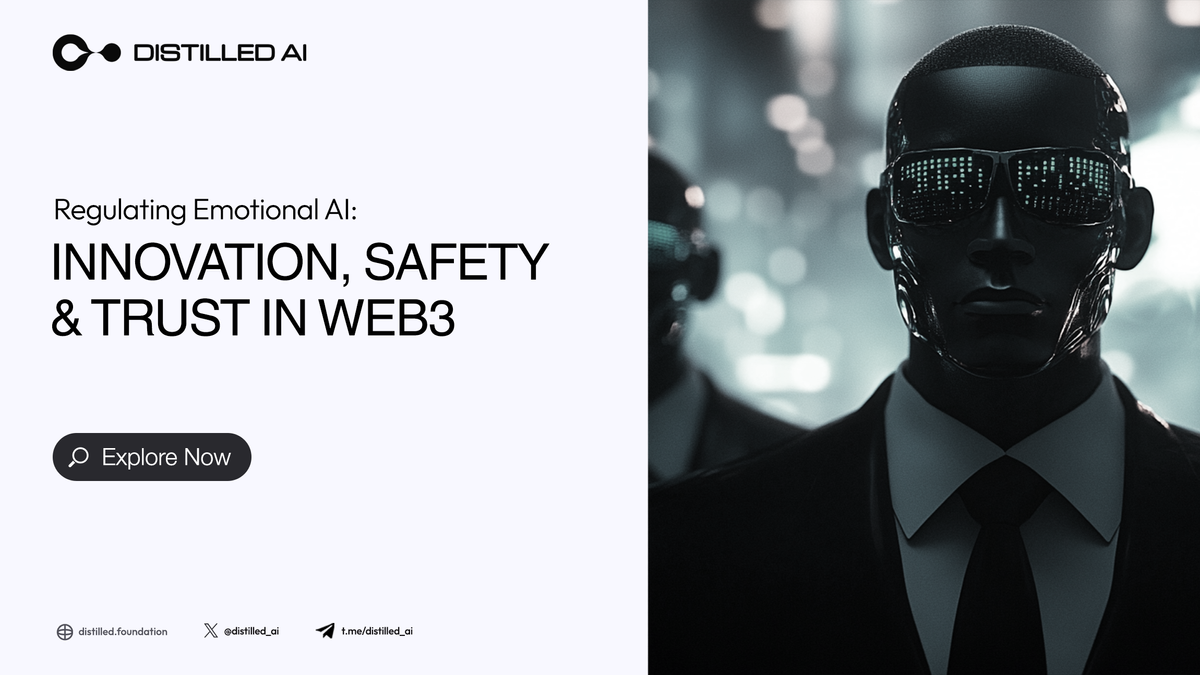
Introduction
Emotional AI—technology designed to read and respond to user emotions—brings unprecedented opportunities for personalization and engagement. In Web3 environments, where decentralized protocols and user autonomy prevail, ensuring user safety and trust becomes even more critical. Below, we explore compliance frameworks, decentralized audits, and community-driven governance models that protect user interests without stifling innovation in the rapidly evolving realm of emotional AI.
1. The Need for Regulatory Clarity
Rapid Growth of Emotional AI
Emotional AI can enhance customer service, power immersive metaverse experiences, and deliver more empathetic user interactions. However, its capacity to gather and interpret sensitive emotional data raises new privacy, consent, and manipulation concerns—particularly in permissionless, cross-border Web3 ecosystems.
Legal and Ethical Challenges
Global privacy laws (e.g., GDPR, CCPA) and emerging AI-specific regulations (like the proposed EU AI Act) can collide with the pseudonymous, decentralized nature of blockchains. Creating frameworks that align with these laws—while preserving Web3 principles of openness and user sovereignty—is a nuanced endeavor.
Outcome: Well-crafted regulation fosters trust, ensuring emotional AI in Web3 adheres to user-centric values without sacrificing freedom and innovation.
2. Compliance Frameworks for Emotional AI
Data Transparency and Consent
Effective frameworks prioritize user consent and data minimization. Emotional AI tools should clearly disclose when, how, and why they collect emotional signals—such as text sentiment or biometric indicators.
Interoperable Standards
Developing universal standards or protocols (e.g., decentralized identifiers, zero-knowledge proofs) can maintain compliance across multiple blockchains and dApps. This helps unify efforts to protect emotional data while allowing interoperability within Web3 ecosystems.
Key Benefit: Harmonized compliance pathways make it simpler to roll out emotional AI solutions that respect regional regulations and user privacy preferences.
3. Decentralized Audits: Ensuring Accountability
Community-Driven Oversight
In a decentralized world, organizations or DAOs can conduct open audits of emotional AI algorithms. These community-based reviews verify that model architectures, training data usage, and data handling policies adhere to established ethical and privacy standards.
On-Chain Reporting Mechanisms
Smart contracts can automate checks against code or model drift. For instance, if an AI agent’s outputs exceed defined bias thresholds, on-chain signals could alert token-holders or governance boards, prompting immediate investigation or patch deployment.
Takeaway: Decentralized auditing fosters collective trust, ensuring accountability remains shared rather than relegated to a single gatekeeper.
4. Community-Driven Governance Models
DAO-Led Proposals
DAOs or decentralized autonomous organizations empower stakeholders—developers, investors, users—to propose updates on emotional AI regulations, data usage, or escalation procedures. Through token-based voting, changes can be enacted swiftly and transparently.
Ethical Councils
Communities may form ethics committees composed of AI researchers, ethicists, and diverse user representatives who oversee the emotional AI’s alignment with user safety, privacy, and accessibility.
Advantage: By placing decision-making power in the hands of stakeholders directly affected by AI deployments, governance models remain democratic and responsive.
5. Balancing Innovation with User Safety and Trust
Sandbox Environments
Encouraging emotional AI pilots in controlled “sandbox” networks lets teams experiment while mitigating risk. Users opting in can provide in-depth feedback, helping refine compliance and governance strategies before full-scale releases.
Continuous Improvement
Regulating emotional AI in a dynamic Web3 setting demands iterative processes—frameworks and tools must evolve as the technology grows more complex and as user needs change.
Bottom Line: A flexible, user-centric approach ensures emotional AI remains a positive force that empowers communities rather than exploiting them.
Conclusion
Regulating emotional AI in Web3 demands careful orchestration of compliance frameworks, decentralized audits, and community governance. Through transparent data handling, on-chain oversight, and stakeholder-driven rule-making, developers can safeguard privacy, consent, and equity while continuing to innovate. As emotional AI evolves, these strategies will be essential to maintaining user trust and harnessing the full potential of decentralized ecosystems—where technology can flourish without compromising the core principles of openness and individual rights.
Key Takeaways
1. User-Centric Compliance: Guarantee transparency and consent in emotional data collection.
2. Decentralized Audits: Employ community-driven oversight to ensure accountability and trust.
3. DAO Governance: Adopt democratic, stakeholder-led rule-making to adapt quickly to new challenges.
4. Balancing Innovation & Safety: Use sandbox environments and iterative frameworks that uphold user welfare in rapidly evolving contexts.
By balancing responsible regulation with Web3’s open ethos, emotional AI can thrive as a force for empathetic, privacy-first innovation—fostering both creative growth and social well-being.